In today’s technological world, data drives every aspect of business processes and decisions. An entire field of study, applied metrology, is based around the collection, standardization, and validation of data. Whether your application is scientific, industrial, or commercial, high-quality, accurate data is the most critical component of the work you do. When it comes to measuring and collecting 3D data, accuracy is key, as is the reduction of possible error in that data.
In order to talk about data accuracy, we must discuss error. Error is the mathematical difference between the actual data points we collect and the values we intend to collect. When using an articulated arm such as the MicroScribe portable CMM for collecting measurement data, there are three basic things we need to know to determine how to prevent error: where it comes from or the source of the error, the quantity of error, and the type or quality of error.
Quantity of Error
It can often be difficult to determine these factors. The quantity of error from a device such as an articulated portable CMM can be extremely small, even as small as 0.0005”. Influences in the environment or system around the device can impact the error of the measurement, so reducing an error below a thousandth of an inch has very little effect on the reality of the error that occurs when we are measuring an object. This is because error from other physical factors, like thermal expansion of the device due to fluctuating temperature, jittering of the device from user input, etc. begins to have a greater impact.
Reducing Error
To reduce error in these cases, you must be able to qualify the error. For example, with error that is Gaussian in nature (a randomly distributed error), with a large enough sample selection, you can use statistics to reduce that error to a much smaller impact on the final XYZ point. Much of the Gaussian error that occurs represents impacts such as jitter from a person, vibration within the environment, or in the case of a bridge CMM, the mechanical hysteresis, where the physical effect on the CMM lags slightly behind its motion. On an articulated arm, each joint’s error may be Gaussian, but the total hysteresis may not be.
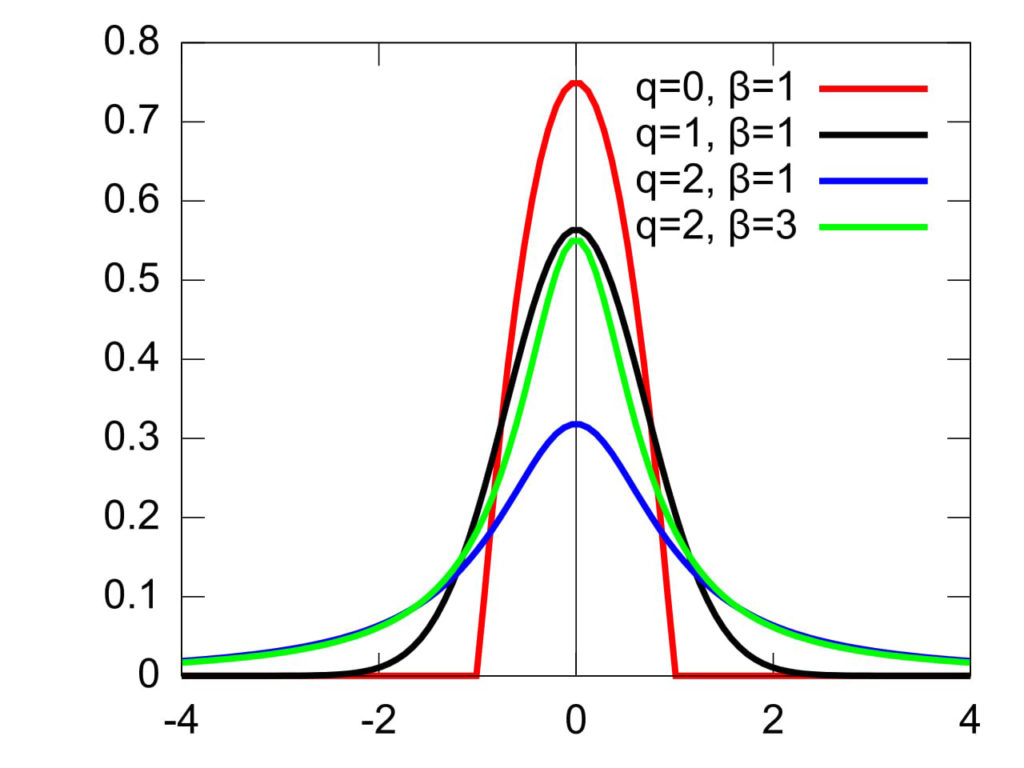
Other errors that cannot be corrected statistically include transcription errors, such as losing decimal points or user mistyping. In this case, you must instill a process to remove the error, for example limiting the way data are transcribed or moved around by consistently using the same software for both measurement and analysis. This removes the possibility of transcription errors by saving out, or reading into a file, or having the user type in known numbers. Avoiding this type of error is key since analyzed data sets that contain transcription errors become suspect. Unfortunately, certain errors are not recoverable. For example, once cosine errors occur via rounding or incorrect processing in geometric rotations, those are not recoverable errors.
To be more specific, in forensic anthropology, many researchers may be taking measurements in a variety of different environments. In this case, you might measure a skull and compare that measurement against that of a colleague, but once the measurement errors are made, it is impossible to remove those errors – you must completely retake the measurements. By utilizing software with built-in checks and balances, remaining consistent with software use, and avoiding manual inputs, you can greatly reduce the error in this type of measurement.
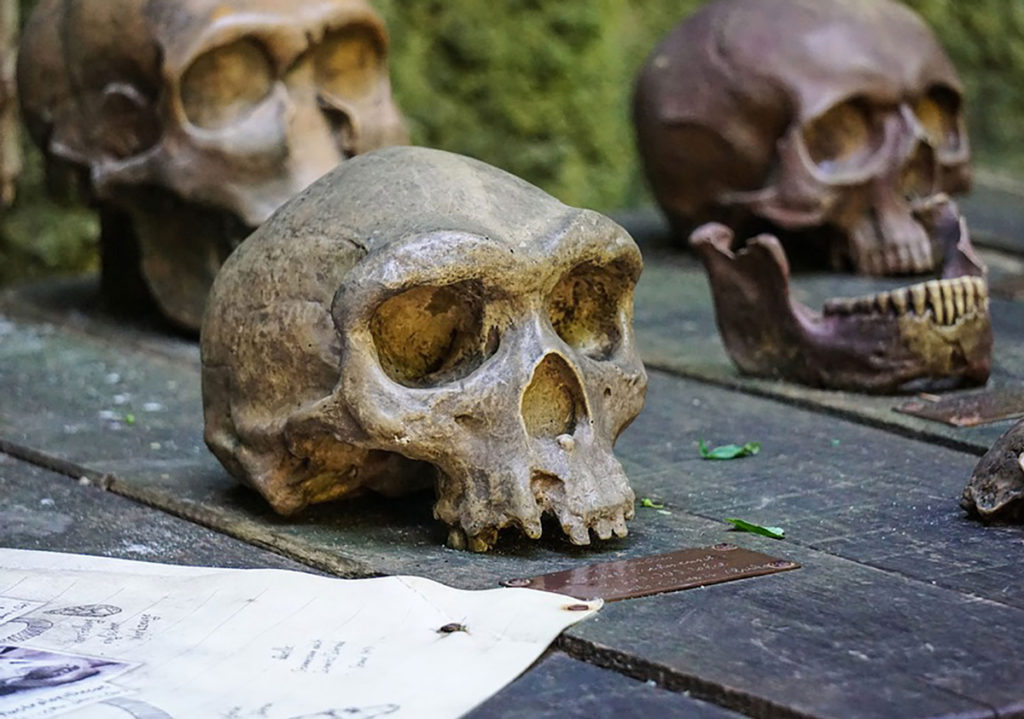
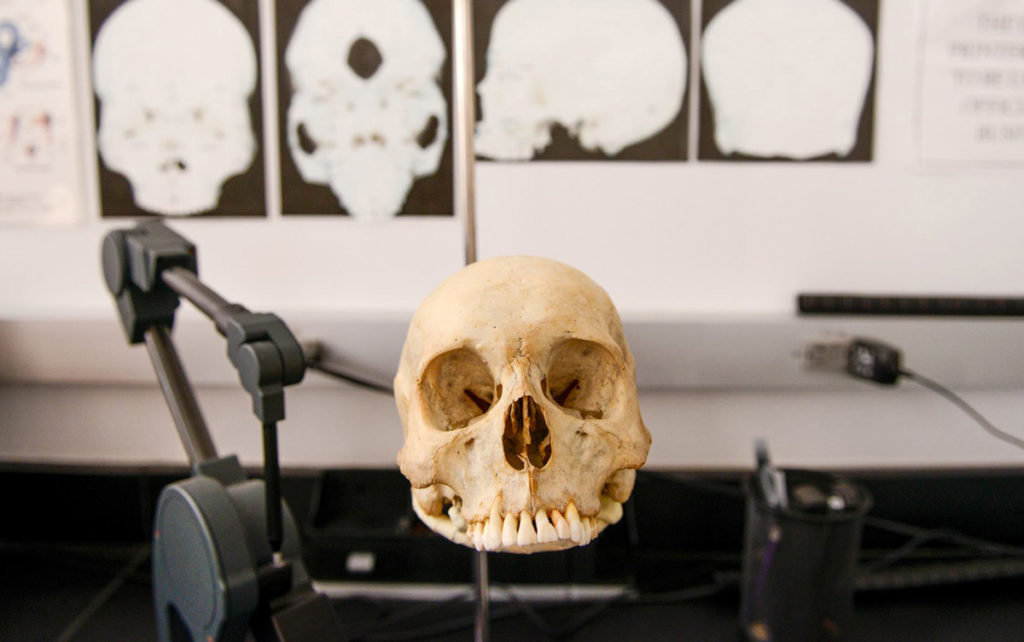
Precision vs. Accuracy
Another important concept when understanding errors in data collection is the distinction between accuracy and precision. Precision is a smaller variation between your sample set, while accuracy is how closely your measurement adheres to the actual value. For example, in archery, precision is how closely grouped the arrows are on the target, while accuracy is how close the arrows are to the bullseye. For measurement with CMMs, both are important; the level of importance applied to either depends on what answer you are looking for.
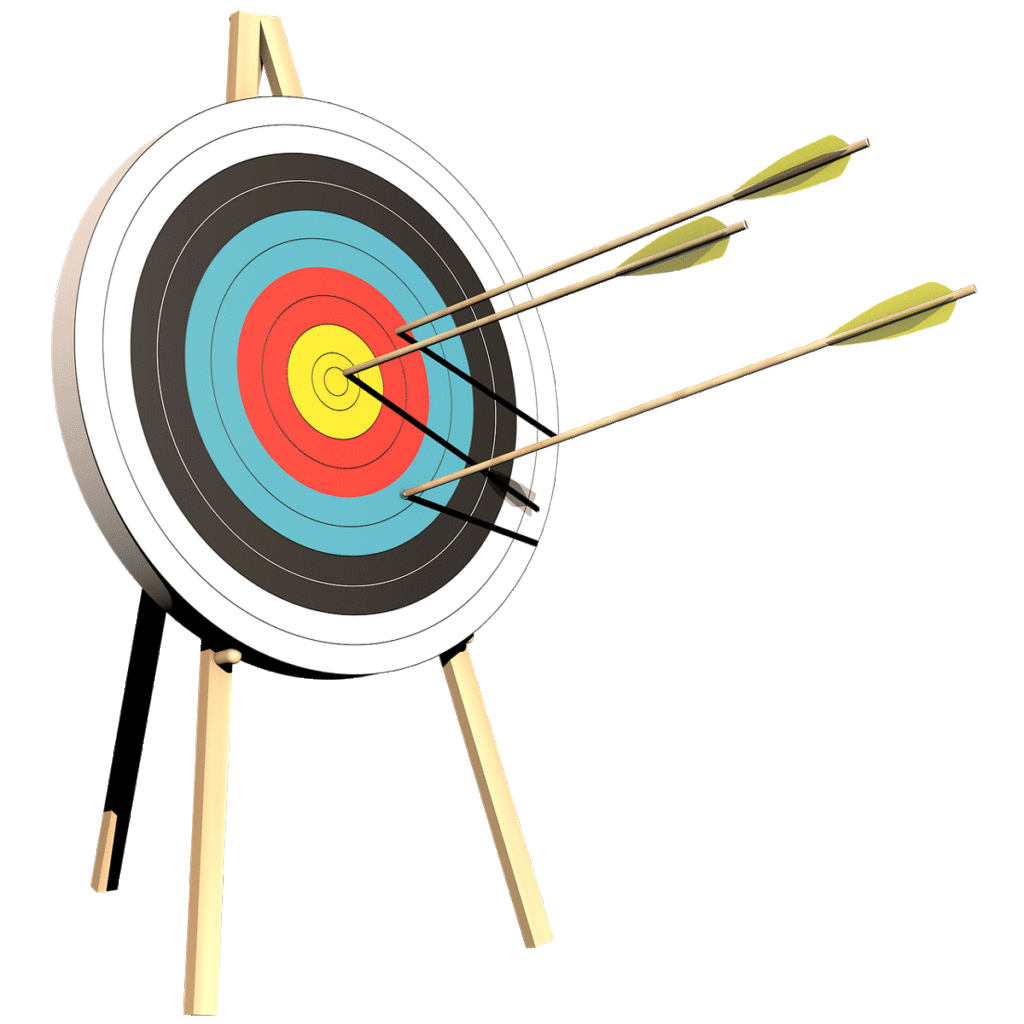
In reverse engineering, variation in manufacturing of the CMM or damage from use will impact error in the precision of the CMM used to measure the object. When trying to measure along a line on the manufactured object, if there is variation in the surface quality, and the difference is a larger percentage of the angle than what you are measuring for, you may end up with faulty data. Fitting a plane across the entire object may provide a general idea of where the surface is. In the end, the process of approach becomes more critical than the error of the device.
Repeatability vs. Reproducibility
Another fundamental concept to understand when reducing error in data measurement is the difference between reproducibility and repeatability. Repeatability is being able to find the same answer within the same environment, while reproducibility is when someone else can follow your process and get the same answer. In the anthropology case mentioned above, an example of repeatability would be that of one scientist measuring points multiple times; if the answers agree, then the measurements were likely correct. However, if there is disagreement in the data, and it is off by more than the margin of error of the device, then there is an error in repeatability.
Reproducibility, however, would be best represented by utilizing the same tools and method in a different environment. If the method is reliable and error-free, the data should be the same. An additional factor is intra-user and inter-user reproducibility – being able to reproduce the results with the same user and with a different user, respectively. For peer-reviewed studies, inter-user reproducibility is the gold standard. Good science relies on other users being able to follow the same process with the same tools and to be able to produce the same set of data.
If your physical environment is relatively stable, you should be able to produce an analog of the same data set as someone else in a stable environment. Though the two environments may differ in environmental factors such as temperature, humidity, etc., those differences can be accounted for and calculated, such as by statistical sampling. There will always inherently be noise in the system; as long as you are aware of that, it is possible to minimize error.
Ultimately, that is one of the strengths of the MicroScribe portable CMM. It is less impacted by user-approached measurement and environmental factors because those environmental variables are a small enough percentage that they do not have an effect in any but the most extreme environments. User error and bad assumptions are more likely to have an impact on error, so building a process to bring attention and awareness to those assumptions will assist in reducing error and providing a better outcome for the measurement.
If you like this article, try Sparse vs. Dense Data by Tom Welsh.
Copyright© 2021 Revware Inc. All rights reserved. Reuse and reprint allowed only with permission by Revware Inc., and with appropriate acknowledgments.
About Revware
Revware provides high, quality, simple to use, innovative, cost-effective modeling solutions to solve your design, metrology, and engineering problems.